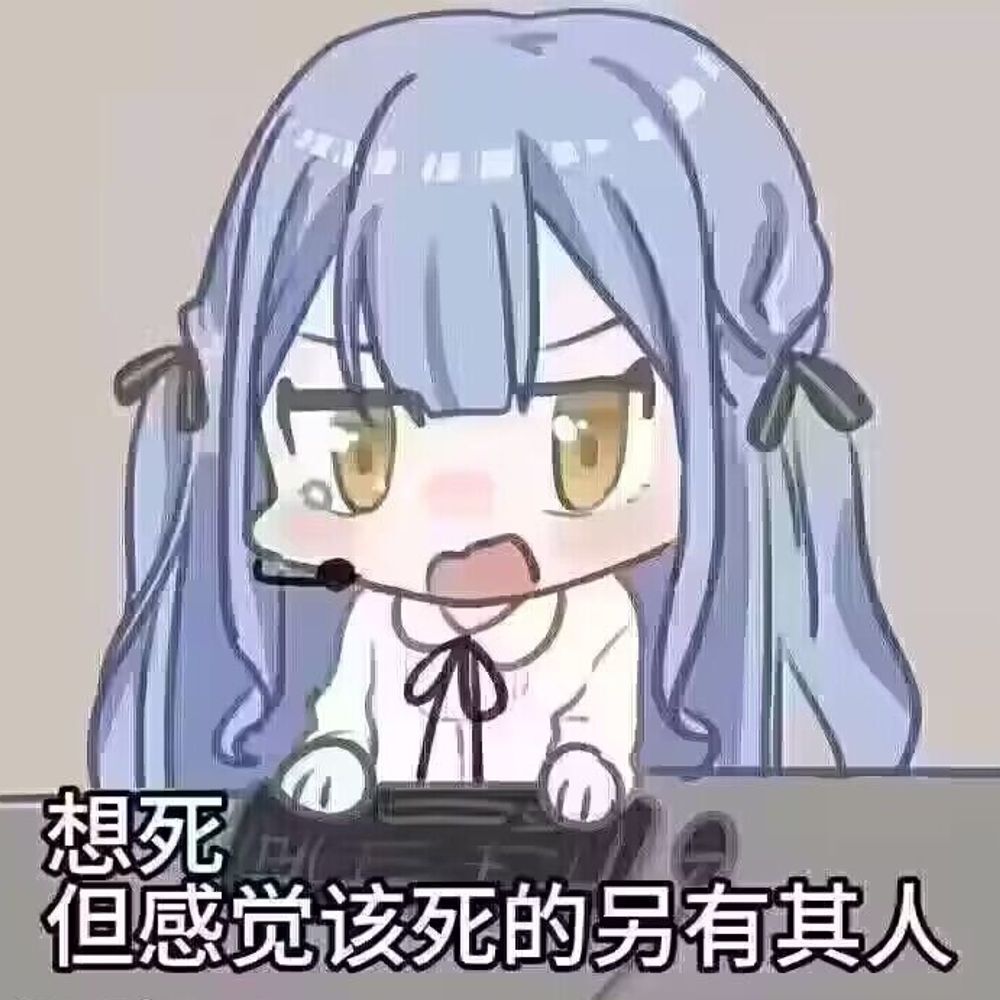
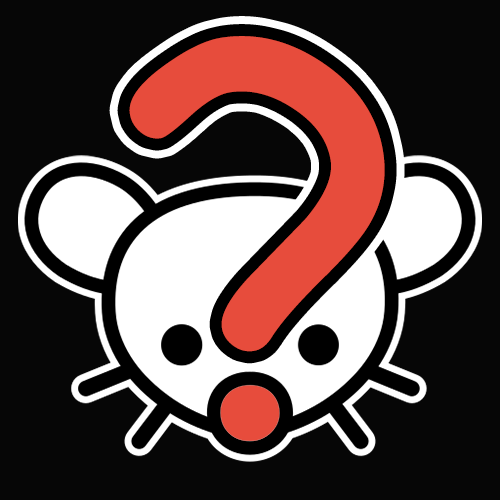
China does tax the rich but they also have an additional system of “voluntary donations.” For example, Tencent “volunteered” to give up an amount that is about 3/4th worth of its yearly profits to social programs.
I say “voluntary” because it’s obviously not very voluntary. China’s government has a party cell inside of Tencent as well as a “golden share” that allows it to act as a major shareholder. It basically has control over the company. These “donations” also go directly to government programs like poverty alleviation and not to a private charity group.
That’s literally China’s policies. The problem is most westerners are lied to about China’s model and it is just painted it as if Deng Xiaoping was an uber capitalist lover and turned China into a free market economy and that was the end of history.
The reality is that Deng Xiaoping was a classical Marxist so he wanted China to follow the development path of classical Marxism (grasping the large, letting go of the small) and not the revision of Marxism by Stalin (nationalizing everything), because Marxian theory is about formulating a scientific theory of socioeconomic development, so if they want to develop as rapidly as possible they needed to adhere more closely to Marxian economics.
Deng also knew the people would revolt if the country remained poor for very long, so they should hyper-focus on economic development first-of-foremost at all costs for a short period of time. Such a hyper-focus on development he had foresight to predict would lead to a lot of problems: environmental degradation, rising wealth inequality, etc. So he argued that this should be a two-step development model. There would be an initial stage of rapid development, followed by a second stage of shifting to a model that has more of a focus on high quality development to tackle the problems of the previous stage once they’re a lot wealthier.
The first stage went from Deng Xiaoping to Jiang Zemin, and then they announced they were entering the second phase under Hu Jintao and this has carried onto the Xi Jinping administration. Western media decried Xi an “abandonment of Deng” because western media is just pure propaganda when in reality this was Deng’s vision. China has switched to a model that no longer prioritizes rapid growth but prioritizes high quality growth.
One of the policies for this period has been to tackle the wealth inequality that has arisen during the first period. They have done this through various methods but one major one is huge poverty alleviation initiatives which the wealthy have been required to fund. Tencent for example “donated” an amount worth 3/4th of its whole yearly profits to government poverty alleviation initiatives. China does tax the rich but they have a system of unofficial “taxation” as well where they discretely take over a company through a combination of party cells and becoming a major shareholder with the golden share system and then make that company “donate” its profits back to the state. As a result China’s wealth inequality has been gradually falling since 2010 and they’ve become the #1 funder of green energy initiatives in the entire world.
The reason you don’t see this in western countries is because they are capitalist. Most westerners have an mindset that laws work like magic spells, you can just write down on a piece of paper whatever economic system you want and this is like casting a spell to create that system as if by magic, and so if you just craft the language perfectly to get the perfect spell then you will create the perfect system.
The Chinese understand this is not how reality works, economic systems are real physical machines that continually transform nature into goods and services for human conception, and so whatever laws you write can only meaningfully be implemented in reality if there is a physical basis for them.
The physical basis for political power ultimately rests in production relations, that is to say, ownership and control over the means of production, and thus the ability to appropriate all wealth. The wealth appropriation in countries like the USA is entirely in the hands of the capitalist class, and so they use that immense wealth, and thus political power, to capture the state and subvert it to their own interests, and thus corrupt the state to favor those very same capital interests rather than to control them.
The Chinese understand that if you want the state to remain an independent force that is not captured by the wealth appropriators, then the state must have its own material foundations. That is to say, the state must directly control its own means of production, it must have its own basis in economic production as well, so it can act as an independent economic force and not wholly dependent upon the capitalists for its material existence.
Furthermore, its economic basis must be far larger and thus more economically powerful than any other capitalist. Even if it owns some basis, if that basis is too small it would still become subverted by capitalist oligarchs. The Chinese state directly owns and controls the majority of all its largest enterprises as well as has indirect control of the majority of the minority of those large enterprises it doesn’t directly control. This makes the state itself by far the largest producer of wealth in the whole country, producing 40% of the entire GDP, no singular other enterprise in China even comes close to that.
The absolute enormous control over production allows for the state to control non-state actors and not the other way around. In a capitalist country the non-state actors, these being the wealth bourgeois class who own the large enterprises, instead captures the state and controls it for its own interests and it does not genuinely act as an independent body with its own independent interests, but only as the accumulation of the average interests of the average capitalist.
No law you write that is unfriendly to capitalists under such a system will be sustainable, and often are entirely non-enforceable, because in capitalist societies there is no material basis for them. The US is a great example of this. It’s technically illegal to do insider trading, but everyone in US Congress openly does insider trading, openly talks about it, and the records of them getting rich from insider training is pretty openly public knowledge. But nobody ever gets arrested for it because the law is not enforceable because the material basis of US society is production relations that give control of the commanding heights of the economy to the capitalist class, and so the capitalists just buy off the state for their own interests and there is no meaningfully competing power dynamic against that in US society.